일 | 월 | 화 | 수 | 목 | 금 | 토 |
---|---|---|---|---|---|---|
1 | 2 | 3 | 4 | 5 | ||
6 | 7 | 8 | 9 | 10 | 11 | 12 |
13 | 14 | 15 | 16 | 17 | 18 | 19 |
20 | 21 | 22 | 23 | 24 | 25 | 26 |
27 | 28 | 29 | 30 |
- UBAR: Towards Fully End-to-End Task-Oriented Dialog System with GPT-2
- 뉴텝스 400
- RuntimeError: DataLoader worker (pid(s) ) exited unexpectedly
- NLP 논문 리뷰
- attention 설명
- Exploring the Limits of Transfer Learning with a Unified Text-to-Text Transformer 리뷰
- BERT: Pre-training of Deep Bidirectional Transformers for Language Understanding 리뷰
- 정책기반 agent
- Evaluate Multiwoz
- 바닥부터 배우는 강화 학습
- BERT 사용방법
- ImageNet Classification with Deep ConvolutionalNeural Networks 리뷰
- Multi Task Learning Objectives for Natural Language Processing 리뷰
- The Natural Language Decathlon:Multitask Learning as Question Answering
- Zero-shot Generalization in Dialog State Tracking through GenerativeQuestion Answering
- Attention Is All You Need
- BERT: Pre-training of Deep Bidirectional Transformers for Language Understanding 논문리뷰
- CNN 논문리뷰
- 길찾기
- Exploring the Limits of Transfer Learning with a Unified Text-to-Text Transformer
- Multi Task Learning Objectives for Natural Language Processing
- BERT란
- MMTOD
- TOD 논문리뷰
- BART 논문리뷰
- 다양한 모듈에서 log쓰기
- A Neural Attention Model for Abstractive Sentence Summarization
- T5 논문 리뷰
- hugging face tokenizer에서 special case 추가하기
- Attention Is All You Need 리뷰
- Today
- Total
one by one ◼◻◼◻
[Summarazation] A Neural Attention Model for Abstractive Sentence Summarization 본문
[Summarazation] A Neural Attention Model for Abstractive Sentence Summarization
JihyunLee 2021. 11. 3. 22:21논문 링크 : https://arxiv.org/abs/1509.00685
A Neural Attention Model for Abstractive Sentence Summarization
Summarization based on text extraction is inherently limited, but generation-style abstractive methods have proven challenging to build. In this work, we propose a fully data-driven approach to abstractive sentence summarization. Our method utilizes a loca
arxiv.org
2015 년에 나온 논문으로 인용수가 무려 2000 회가 넘는 논문입니다. 지금은 Summarization에 transformer 모델을 활용해서 하고 있지만 2015년에는 신경망을 적용하여 summarization을 시도한 것이 거의 없었다고 합니다. 특히 attention 기법또한 적용하여 abstractive한 summarization을 연구한 논문입니다.
일단 summarization의 목적은
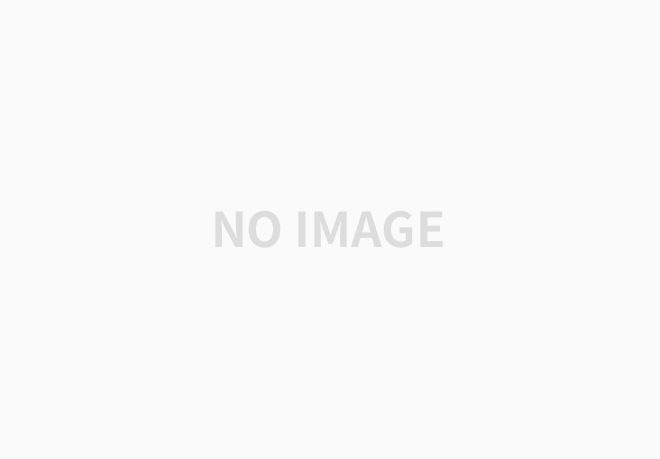
s 가 scoring function이라고 했을 때, x가 들어갔을 때 가장 그럴듯한 y 를 만들어 내는것이 목적입니다.
사용한 모델은 NNLM모델입니다.
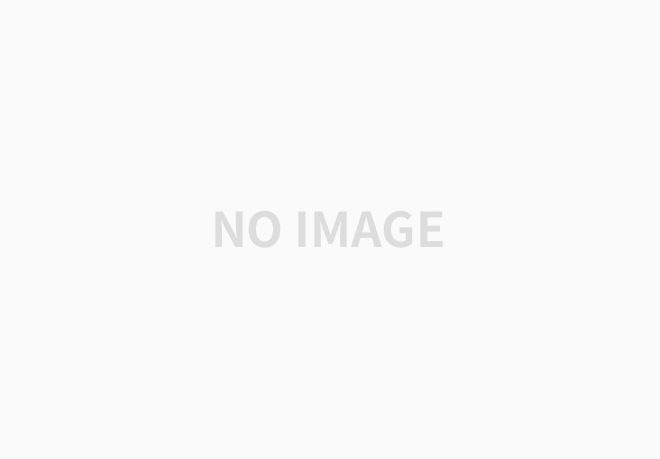
NNLM모델은 아래의 식을 가지고 있으며
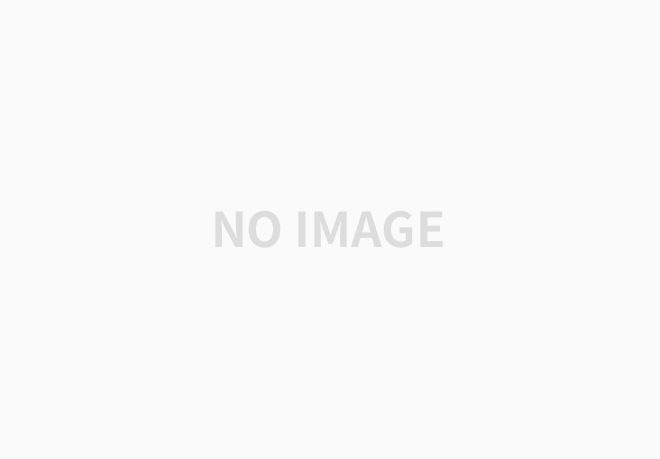
E은 encoding을, U, V, W를 학습가능한 parameter로 가지며, exp(Vh + Wenc(x,yc)) 의 결과로 각 전체 단어 벡터에서 '그 단어가 문장의 다음단어로 들어갈' 확률값이 나오게 됩니다.
논문에서는 Encoding으로 Bag of words 인코딩과 Convolution encoder, Attention based encoder를 사용하였는데 그중에서 가장 성능이 좋았던 encoding은 attention based encoding이었습니다.
2) 바다나우 어텐션(Bahdanau Attention)
지난 챕터에서 어텐션 메커니즘의 목적과 어텐션 메커니즘의 일종인 닷 프로덕트 어텐션(루옹 어텐션)의 전체적인 개요를 살펴보고, 마지막에 표를 통해 그 외에도 다양한 어텐션 ...
wikidocs.net
위는 제가 읽어본 attention설명 중 가장 잘 된 글인것 같아서 링크로 남겨두겠습니다.
논문은 위 NNLM구조를 이용해서 summary를 generating하고 beam search를 통해서 몇가지 좋은 선택지를 가려 냅니다. (간단히 말하자면 beam search는 괜찮은 후보 여러개를 계속해서 가지치기 하듯 만들어 나가서 최종적으로 가장 좋은 선택지를 선택하는 문제입니다.)
논문은 DUC-2004 대회에서 가장 좋은 성능을 달성했습니다.